Fei-Fei Li is the director of the Computer and Human Vision Lab within the legendary Stanford Artificial Intelligence Laboratory. While her research interests and breakthrough contributions to the field are wide-ranging, I want to focus briefly on a 2009 study she and her colleagues did at Princeton, before Dr. Li's selection to head the prestigious Stanford Vision Lab. "Towards Total Scene Understanding: Classification, Annotation and Segmentation in an Automatic Framework" is a remarkable project and representative of the kind of machine-learning image recognition capabilities envisioned at "serious play" in the 'Seeing Eye Child' Robot Adoption Agency game.
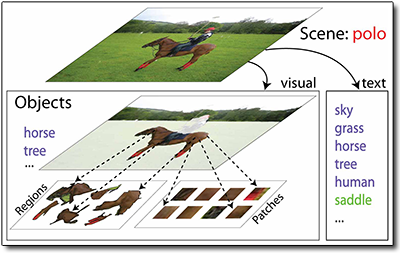
Researchers like Dr. Li are creating machine-learning programs that can effectively 'look' at a previously unseen image and not just find objects within the image but interpret the full scene. This example graphic from the project's summary shows how a total scene is considered as a comprehensive model that incorporates both top-down context, e.g., a polo match, along with both visual and textual (tag) elements. For Dr. Li and associates' study, the tags and images were drawn from Flickr – yes, the same popular image-sharing site where the British Library released its 1-million-plus public domain image collection – and the end-to-end automatic machine-learning/scene-recognition process is generally described as shown in the following 3-step workflow diagram from the study.
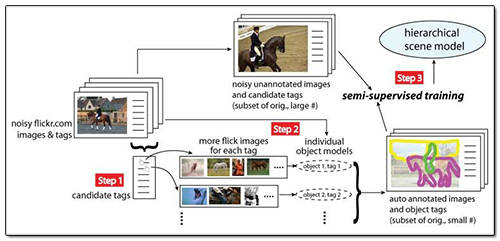
The results of this machine-learning strategy are both remarkable and encouraging for our game design requirements. Given a 'seed' set of curated images with text label 'hints' and a representative collection of similar-scene images clustered by the eight targeted sports categories, the researchers' automated process does a remarkable job of finding and labeling these elements in new unseen images, producing results such as this test image which has been correctly recognized as a polo scene with horse and rider, trees, and grass:
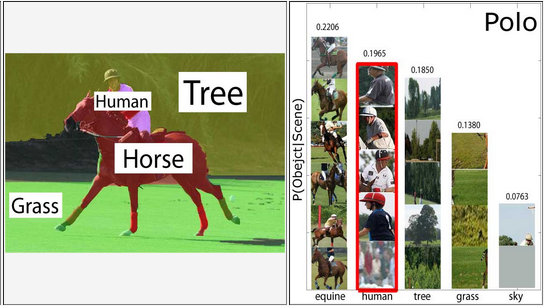
While Dr. Li and associate's strategy is particularly robust and comprehensive, their paper cites 22 additional studies in four broad areas of CV/AI research that tackle the full scene understanding challenge:
- Image understanding using contextual information
- Machine translation between words and images
- Simultaneous object recognition and segmentation
- Learning semantic visual models from Internet data
So it is safe to say that the domains of computer vision and artificial intelligence (CV/AI) are at a sufficient stage of capability and active research that the kind of 'robot' vision required for our game design is both doable and getting better. If you have any doubts (or better yet, interest to know more), I encourage you to read Dr. Li's project overview, or better yet, the full study PDF and follow links to cited related research.
In the concluding post of this series about the potential for FactMiners to contribute to the "serious fun" at the British Library Image Collection, I'll set some goals and chart a course forward to add the 'Seeing Eye Child' Robot Adoption Agency to the selection of social-learning games to be developed by, and available to, the FactMiners gaming community.